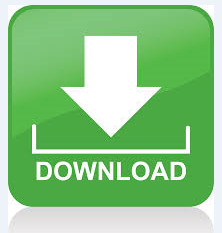
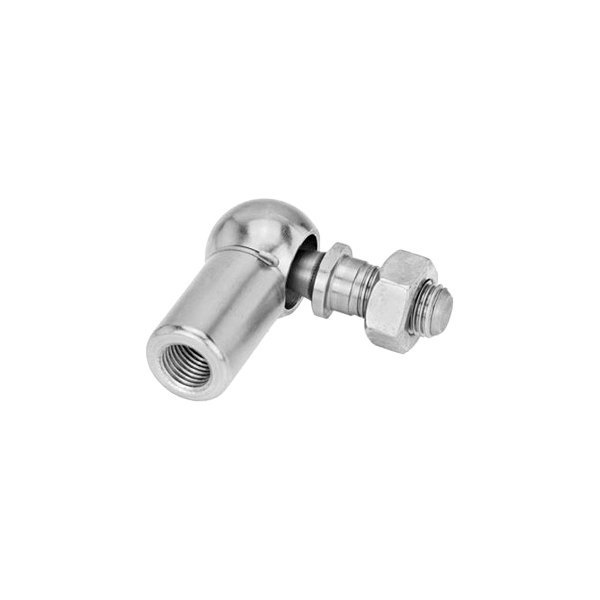
the truck would still be profitable and it would not have gotten sold.
/cdn.vox-cdn.com/uploads/chorus_image/image/17540299/136100201.0.jpg)
You purchased it in its current condition but the guy who had it done, then sold the truck prolly knew damn well it was not right.

The odds of having a program in that thing that is even half-correct, nonetheless right are greatly against you. You need to pull a copy of the program out of that ECM and e-mail it to myself, Mommaburt, or Unilevers, and have it inspected for a second opinion. Sounds like the victim of yet another bad delete program just like 99.99% of everyone else that finds my site after tearing up a few turbo's or blowing ahead gasket or 2. Have boost gauge running at 15 to 28 pyro gauge straight hill and temp goes from 600 to over 1000 in minutes been running below 1000 never above 1100 just put 2nd turbo on please help However need any help with the turbo overheating. From figure 1,intuition suggests (and it can be shown) that the projection issimply given by the normalization of a.(04-08-2019 )Rnttrans Wrote: Just but 2012 KW that was deleted was.not told till afterwards. However, a projectiononto the l 2 -norm ball has a closed-form expression andtherefore turns to be an easy task to perform.

1.x 2(b) l 2 -ballx 1The unit norm ballsx 2(c) l ∞-ballThe problem (1) is a convex optimization which can beefficiently solved in polynomial time. Examples of the three unit norm ballsin R 2 can be shown in Figure 1.x 2(a) l 1 -ballx 1Fig. INTRODUCTIONThe problem of computing the Euclidean projection of avector a ∈ R n onto the unit l p -norm ball can be casted asminimize ‖y −a‖ 2 2subject to ‖y‖ p ≤ 1where p = 1,2 or ∞. With the proposed methodwe are able to solve problems of dimensions in the order ofseveral thousand efficiently.Index Terms-l 1-norm, sparse estimation, autoregressive models,convex optimizationI. Numerical exampleswith synthetic data sets are included. Our approach is useful for sparsesystem identification problems that can be represented as aminimization of a loss function subject to l 1-norm constraints.Sparse autoregressive model estimation is included as an exampleof this problem type, where zeros in autoregressive coefficientsindicates a causality structure of variables. We demonstrate the effectiveness of themethod and compare the results with solving the primal problemby an interior-point method. Themethod involves sorting elements of the vector and performinga linear interpolation. We present anefficient method for the projection, obtained via the dual problemwhich reduces to an optimization with a scalar variable. This can becasted as a simple convex optimization problem. Projection onto an l 1 -norm Ball with Application toIdentification of Sparse Autoregressive ModelsJitkomut SongsiriDepartment of Electrical EngineeringFaculty of Engineering, Chulalongkorn University254 Phayathai Rd., Pathumwan, Bangkok, 10330 ThailandEmail: consider a problem of finding the Euclideanprojection of a vector in R n onto an l 1-norm ball.
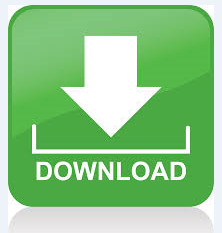